11 Popular Machine Learning / AI Frameworks and Libraries in 2025
In our fresh article, discover the top 11 popular artificial intelligence and machine learning frameworks to build robust and flexible architectures for your AI-powered apps.
In the rapidly evolving landscape of technology, Artificial Intelligence (AI) and Machine Learning (ML) have emerged as revolutionary forces reshaping industries and daily life. These technologies power the behind-the-scenes magic in everything from personal voice assistants to predictive analytics in healthcare.
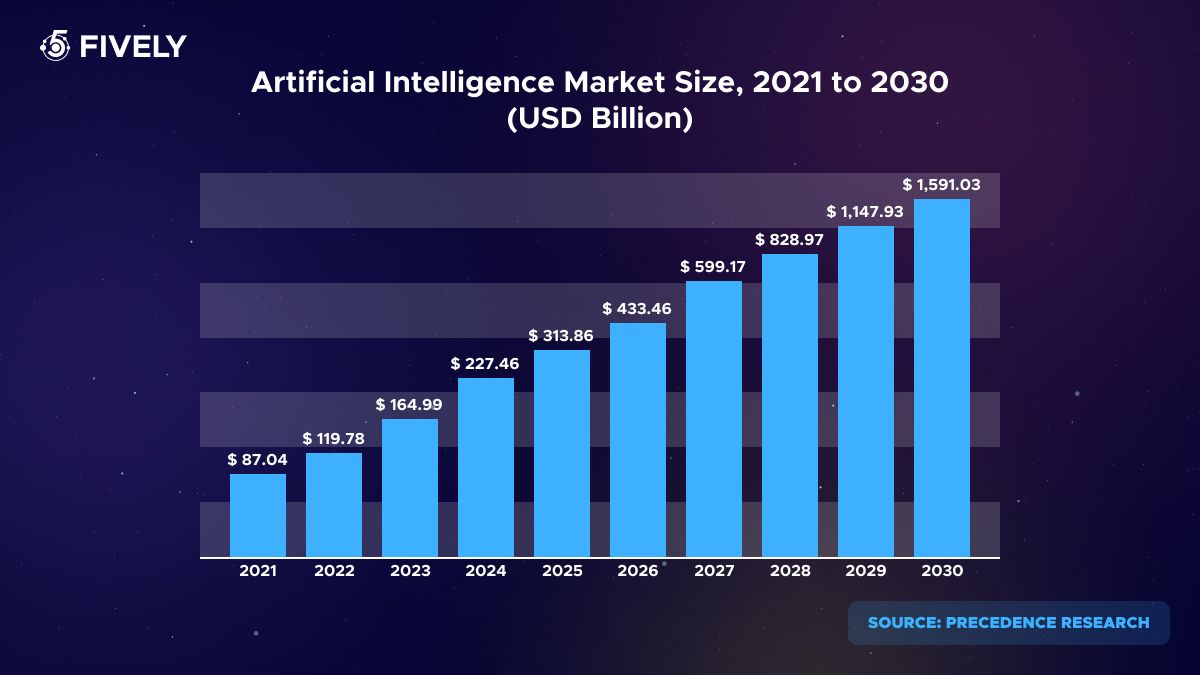
As AI and ML continue to burgeon, an array of tools and frameworks have been developed to aid businesses in harnessing their potential. With countless options available, it can be overwhelming to determine which tools are most apt for specific tasks. Here at Fively, we've compiled a concise list of the top 11 tools and frameworks to guide you in making informed decisions.
Let's journey through each of these indispensable tools with our top ML and AI specialist Andrew Oreshko!
List of AI Frameworks & Tools
TensorFlow
It’s an open-source library for dataflow and differentiable programming developed by the Google Brain team. It's primarily used for machine learning applications and deep neural network research.
Languages: Python, C++, and Java
Platforms: Linux, macOS, Windows, Android, iOS (via TensorFlow Lite)
+ Highly flexible and modular;
+ Excellent community support;
+ Scalable for both small and large applications;
- The steeper learning curve for beginners;
- Some operations can be less intuitive compared to other ml frameworks.
“TensorFlow, as one of the market leaders, is ideally suited for large projects due to a developed ecosystem of products (TensorFlow Hub, TensorFlow Serving, TensorFlow Lite), great community, detailed documentation, and lots of code examples over the internet.”
Sci-kit Learn
A free machine learning library that integrates seamlessly with the Python language. It's suitable for various machine-learning tasks, including classification, regression, and clustering.
Languages: Python
Platforms: Linux, macOS, Windows
+ Comprehensive library for machine learning algorithms;
+ Strong documentation and community support;
+ Ease of use and integration;
- Not tailored for deep learning;
- Doesn't support GPU acceleration.
“Ski-kit Learn, just like Pandas, is very convenient, and has a bunch of ready-made packaged solutions.”
Pandas
A fast, powerful, and flexible open-source data analysis and data manipulation library.
Languages: Python
Platforms: Linux, macOS X, Windows
+ Comprehensive data manipulation tools;
+ Seamless integration with many other data science tools;
+ Strong community support;
- Memory consumption can be high;
- Some operations can be slower than pure NumPy.
“Pandas attracts with its user-friendly interface. However, it should be noted that it can have problems when working with big data science projects (because it loads all the data it works with into RAM). There is a similar technology called Dask, which can parallelize its work - it’s really interesting to see its further development.”
NumPy
A library for the Python language, allowing support for large, multi-dimensional arrays and matrices along with a vast collection of high-level mathematical functions.
Languages: Python
Platforms: Linux, macOS X, Windows
+ Efficient mathematical operations;
+ Broad functionality for numerical tasks;
+ Seamless integration with other libraries;
- Not specifically tailored for machine learning.
“NumPy does all the computational heavy-lifting for working with vectors and matrices. This is needed both in Pandas (it is built on NumPy), and in Scikit-Learn (since it is M-library, here the speed of calculations is important, which is what NumPy gives us).”
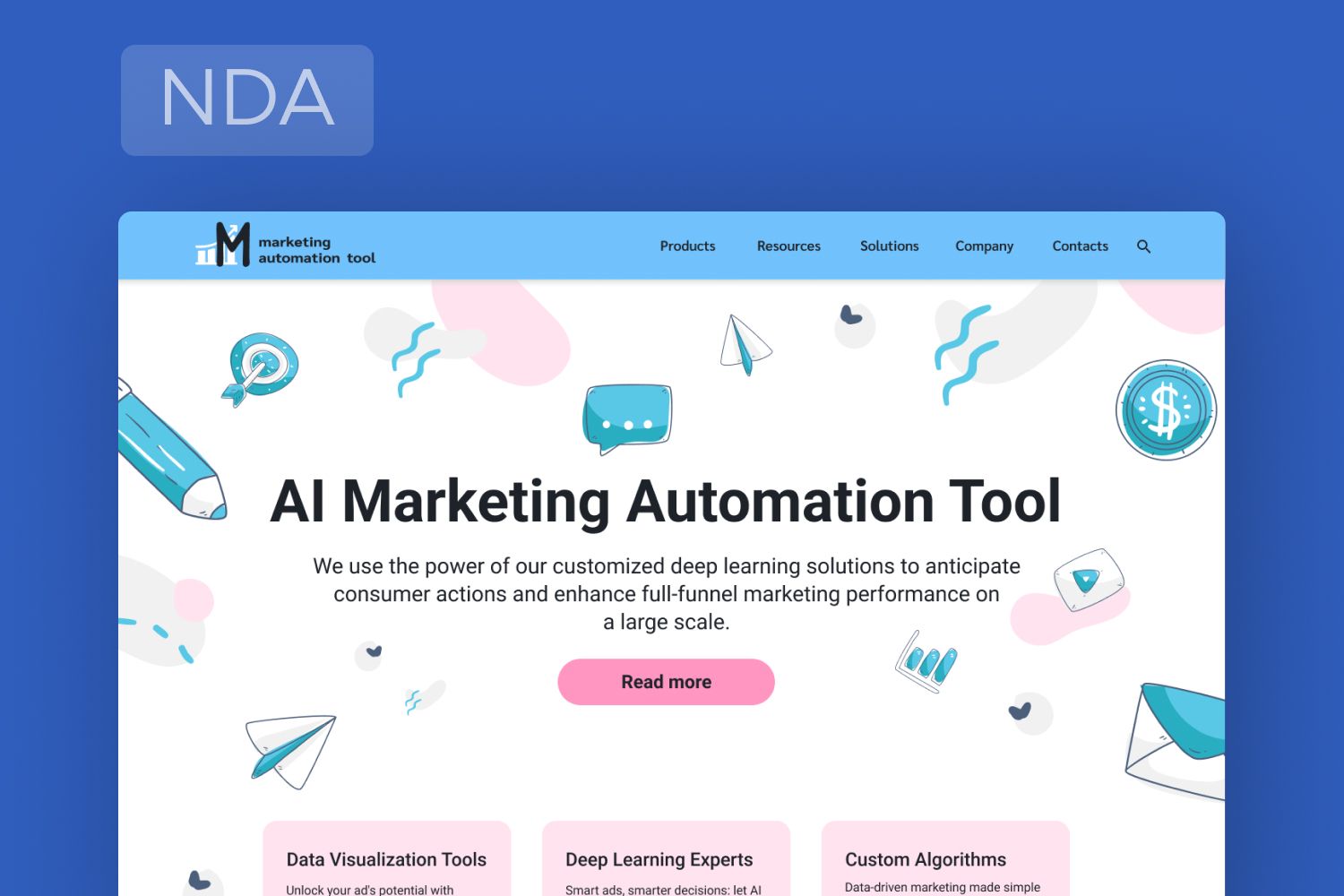
“Sci-kit Learn, Pandas, Numpy - they are the core of ML projects: convenient both for research and for building production-ready systems, they will continue to be used everywhere and to develop further.”
PyTorch
Developed by Facebook's Research lab, it's a dynamic computational graph-based framework often compared to TensorFlow. It allows developers to use GPU acceleration for computations.
Languages: Python, C++
Platfroms: Linux, macOS X, Windows
+ Dynamic computational graph;
+ Intuitive and more pythonic;
+ Strong support for GPU acceleration;
- Slower than some counterparts due to dynamic computation.
“PyTorch, another market leader with a large community and lots of code examples, is ideal for smaller projects and for research.”
Keras
It is an open-source neural network library written in Python. It's known for being user-friendly and modular, acting as an interface for TensorFlow and Theano.
Languages: Python
Platforms: Linux, macOS X, Windows (depends on the backend: TensorFlow, Theano, etc.)
+ User-friendly API;
+ Modular and extendable;
+ Supports multiple backend neural computation engines;
- Performance can sometimes be suboptimal;
- Dependency on TensorFlow or Theano as a backend.
“Keras can run on top of TensorFlow or Theano. Keras is, in fact, a top-level API for these backends (TensorFlow/Theano), which simplifies model development.”
Theano
An open-source numerical computation library that lets developers efficiently define, optimize, and evaluate mathematical expressions.
Languages: Python
Platforms: Linux, macOS X, Windows
+ Highly efficient for numerical tasks;
+ GPU acceleration support;
+ Tight integration with NumPy;
- Development has been halted;
- Not as beginner-friendly as some other options.
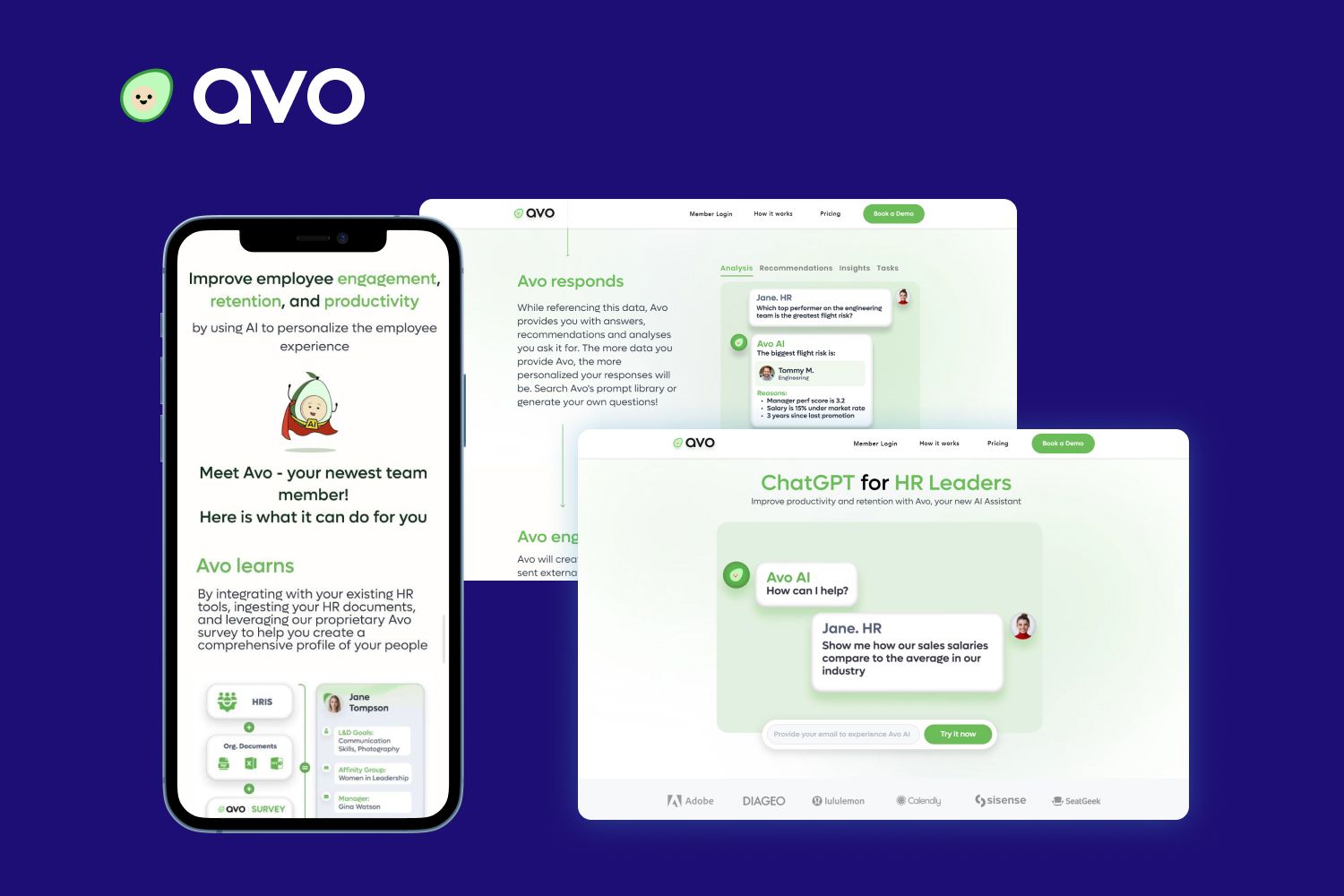
Google ML Kit
A mobile SDK that brings Google's machine learning capabilities to Android and iOS apps, with powerful, yet easy-to-use solutions.
Languages: Java (Android), Swift/Objective-C (iOS)
Platforms: Android, iOS
+ Easy to integrate into mobile apps;
+ Pre-trained models available;
+ Supports custom TensorFlow Lite models;
- Limited to mobile app development;
- Some advanced use cases may need further fine-tuning.
Caffe 2
Now we move on to machine learning and deep learning frameworks. The first one is Caffe 2: an open-source deep learning framework with modularity and speed in mind. Developed by Facebook, it's the successor to the Caffe framework.
Languages: Python, C++
Platforms: Linux, macOS X, Windows, Android, iOS
+ Highly modular and performant;
+ Suitable for mobile deployment;
+ Strong community and support;
- The steeper learning curve for beginners;
- Less popular compared to TensorFlow and PyTorch.
Microsoft CNTK
The Microsoft Cognitive Toolkit, or CNTK for short, is a deep learning framework developed by Microsoft. It is known for its efficiency at scale.
Languages: Python, C++
Platforms: Linux, macOS X, Windows
+ Efficient for large-scale datasets;
+ Strong support for recurrent neural networks (RNNs);
+ High performance and scalability;
+ Less intuitive API compared to some counterparts;
+ Limited community support.
MxNet
A deep learning framework designed for both efficiency and flexibility. It allows developers to mix symbolic and imperative programming.
Languages: Python, C++, Julia, R, Scala, Perl
Platforms: Linux, macOS X, Windows, iOS, Android
+ Supports multi-language APIs;
+ Highly efficient and scalable;
+ Mix of symbolic and imperative programming;
+ Lesser community support compared to TensorFlow and PyTorch;
+ Some parts of the documentation might be lacking.
Top AI Frameworks and Libraries Comparison
With a plethora of options available, it's crucial to choose the right one that aligns with your project requirements, expertise, and platform preferences. In the table below, we've collated the main aspects of some of the leading tools, providing a side-by-side comparison to aid in your decision-making process. Let's get a birds-eye view of these tools and discern the subtle nuances that set each apart.
Popular Machine Learning Frameworks: Final Thoughts
In the realm of Artificial Intelligence, the apprehension surrounding frameworks often stems from the fear of the unknown. Many professionals are wary, thinking that these tools might overshadow or replace their roles. However, solutions like GhatGPT or professional artificial intelligence frameworks like we described above in this article, should be perceived as our allies in building great custom software.
The only question that arises is how to choose the tool that fits your project needs. Here’s how Andrew Oreshko comments on this:
Just as a masterful artist uses brushes to create masterpieces, tools are instruments that, when wielded with expertise, can lead to revolutionary advancements. They complement our human capabilities, harnessing the power of automation and data-driven insights, while we maintain the reins of creativity, strategy, and intuition.
If the world of AI intrigues you, or if you're contemplating leveraging these tools for your business aspirations, look no further. Our seasoned professionals at Fively are adept at integrating AI seamlessly into diverse projects, so don’t hesitate to contact us.
Together, let's craft the future!
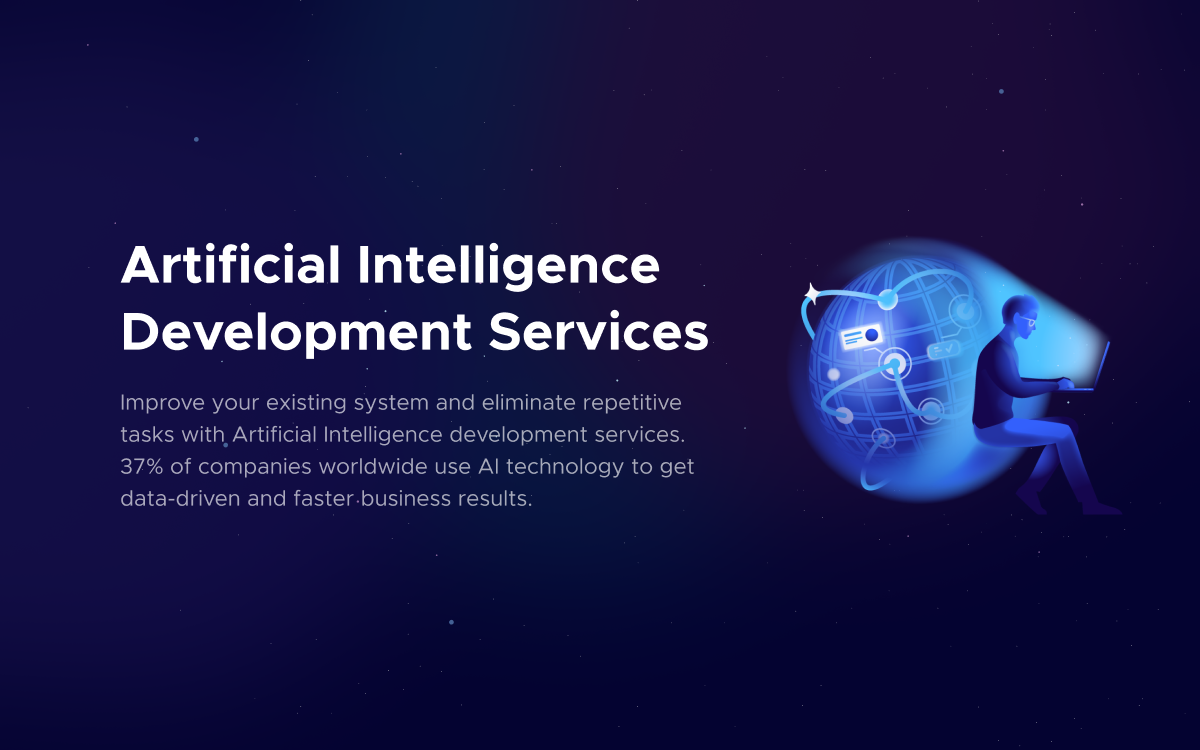
Need Help With A Project?
Drop us a line, let’s arrange a discussion